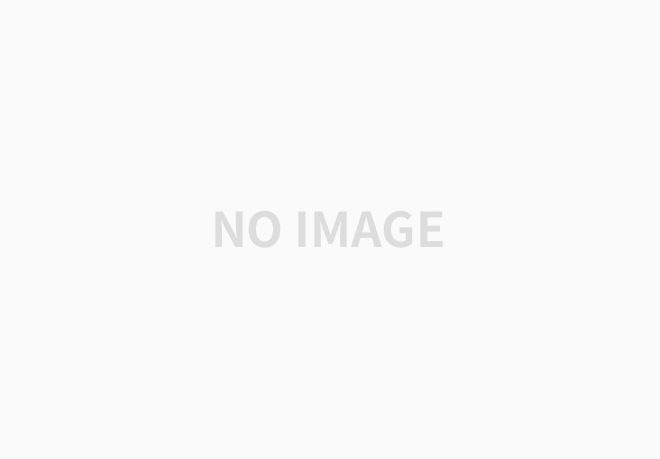
ball_tracker.py
앞 포스팅의 player_tracker과 아주 유사하나, 커스텀한 모델을 사용했다.
박스와 텍스트 색깔을 연두색으로 바꿔주었다.
from ultralytics import YOLO
import cv2
import pickle
class BallTracker:
def __init__(self, model_path):
self.model = YOLO(model_path)
def detect_frame(self, frame):
results = self.model.predict(frame, conf=0.15)[0]
# class_names = results.names
ball_dict = {}
for box in results.boxes:
# track_id = int(box.id.tolist()[0])
result = box.xyxy.tolist()[0]
ball_dict[1] = result
# class_ids = box.cls.tolist()[0]
# det_class_names = class_names[class_ids]
# if det_class_names =="person":
# player_dict[track_id] = result
return ball_dict
def detect_frames(self, frames, read_from_stub=False, stub_path=None):
ball_detections = []
if read_from_stub and stub_path is not None:
with open(stub_path, 'rb') as f:
ball_detections = pickle.load(f)
return ball_detections
for frame in frames:
ball_dict = self.detect_frame(frame)
ball_detections.append(ball_dict)
if stub_path is not None:
with open (stub_path, 'wb') as f:
pickle.dump(ball_detections, f)
return ball_detections
def draw_bboxes(self, video_frames, ball_detections):
output_video_frames=[]
for frame, ball_dict in zip(video_frames, ball_detections):
for track_id, bbox in ball_dict.items():
x1,y1,x2,y2 = bbox
cv2.putText(frame, f"Ball ID: {track_id}", (int(x1), int(y1)-10), cv2.FONT_HERSHEY_COMPLEX_SMALL, 0.9, (0,255,0),2)
cv2.rectangle(frame, (int(x1), int(y1)), (int(x2), int(y2)), (0,255,0),2)
output_video_frames.append(frame)
return output_video_frames
main.py
import cv2
from ultralytics import YOLO
from utils import (read_video, save_video)
from trackers import PlayerTracker, BallTracker
def main():
print("hello")
input_video_path = "input_videos/input_video.mp4"
video_frames = read_video(input_video_path)
#Detect players
player_tracker =PlayerTracker(model_path="yolo11x.pt")
player_detections= player_tracker.detect_frames(video_frames, read_from_stub=True, stub_path = "trackers_stub/player_detection.pkl")
#Detect Ball
ball_tracker = BallTracker(model_path= "models/tennis_ball_best.pt")
ball_detections = ball_tracker.detect_frames(video_frames, read_from_stub=False, stub_path = "trackers_stub/ball_detection.pkl")
#Draw Player Bounding Boxes
output_video_frames = player_tracker.draw_bboxes(video_frames, player_detections)
#Draw Ball Bounding Boxes
output_video_frames = ball_tracker.draw_bboxes(output_video_frames, ball_detections)
#Save the Output Video
save_video(output_video_frames, "output_videos/output.avi")
if __name__ == "__main__":
main()
다음에는 read_from_stub 을 True로 바꿔놓고 진행하면 된다
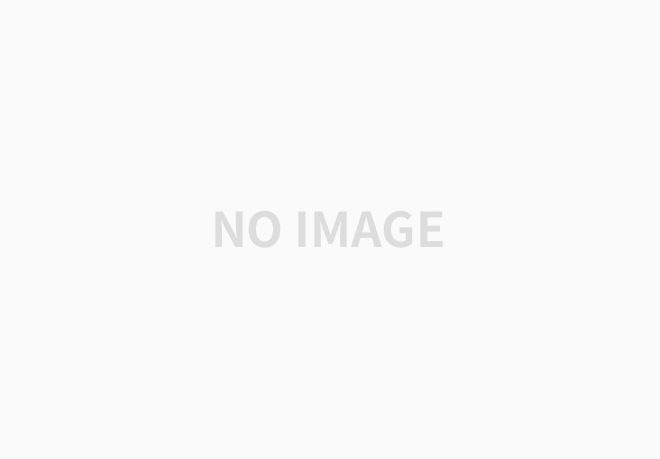
그런데 여기서 한 가지 이슈가 발생한다.
코트 경계선을 공이 지날 때는 제대로 detection되지 않는다는 문제가 발생하는 것이다. 이를 pandas를 가지고 해결해보도록 한다.
ball_tracker.py
import pandas as pd
def interpolate_ball_positions(self, ball_positions):
ball_positions = [x.get(1, []) for x in ball_positions]
df_ball_positions = pd.DataFrame(ball_positions, columns=['x1','y1','x2', 'y2'])
df_ball_positions = df_ball_positions.interpolate()
df_ball_positions = df_ball_positions.bfill()
ball_positions = [{1:x} for x in df_ball_positions.to_numpy().tolist()]
return ball_positions
x.get(1, [])
#x가 딕셔너리라면, 1 키의 값을 가져오고, 없으면 빈 리스트를 반환합니다.
get()는 딕셔너리 메서드로 딕셔너리에서 특정 키를 찾고, 해당 키가 존재하지 않으면 기본값을 반환한다.
예를 들어 x = {1: [10, 20], 2: [30, 40]} 인 상황에서 x.get(1, [])에 대한 해석은 아래와 같다.
1은 딕셔너리에서 찾고자하는 값이기에 해당 키에 대응하는 값을 반환한다. 만약 존재하지 않으면 빈 리스트인 []를 반환한다.
df_ball_positions = df_ball_positions.interpolate()
df_ball_positions = df_ball_positions.bfill()
interpolation은 보간하다는 의미로, 주변의 것으로 지금의 것을 채운다.
다시말해, interpolate() 메서드를 이용하여 볼의 위치가 연속적일 때 두 점 사이의 값들을 예측하여 채워서 볼의 흐름이 자연스럽게 이어지도록 만든다.
bfill()은 뒤에 있는 값으로 나머지를 채운다는 의미이다.
mian.py
#Interpolate ball positions
ball_detections = ball_tracker.interpolate_ball_positions(ball_detections)
'머신러닝 > 영상인식' 카테고리의 다른 글
영상인식 응용 resize, read, hsv, mask, morphology operation, hough, arctan2 (0) | 2025.03.19 |
---|---|
영상인식 기초, pickle 저장하기, yolo11x.pt, player검출하기, __init__.py 역할 (0) | 2025.03.16 |
영상인식 기초 욜로 파이토치 CNN 학습시키기 Resnet50 (0) | 2025.03.16 |
영상인식, roboflow, 데이터라벨링, 가지고 있는 데이터를 이용해 모델 만들기 (1) | 2025.03.09 |
YOLO 기초, 영상 인식, 영상에서 사물인식하기 yolo11x, yolo11n (0) | 2025.03.08 |